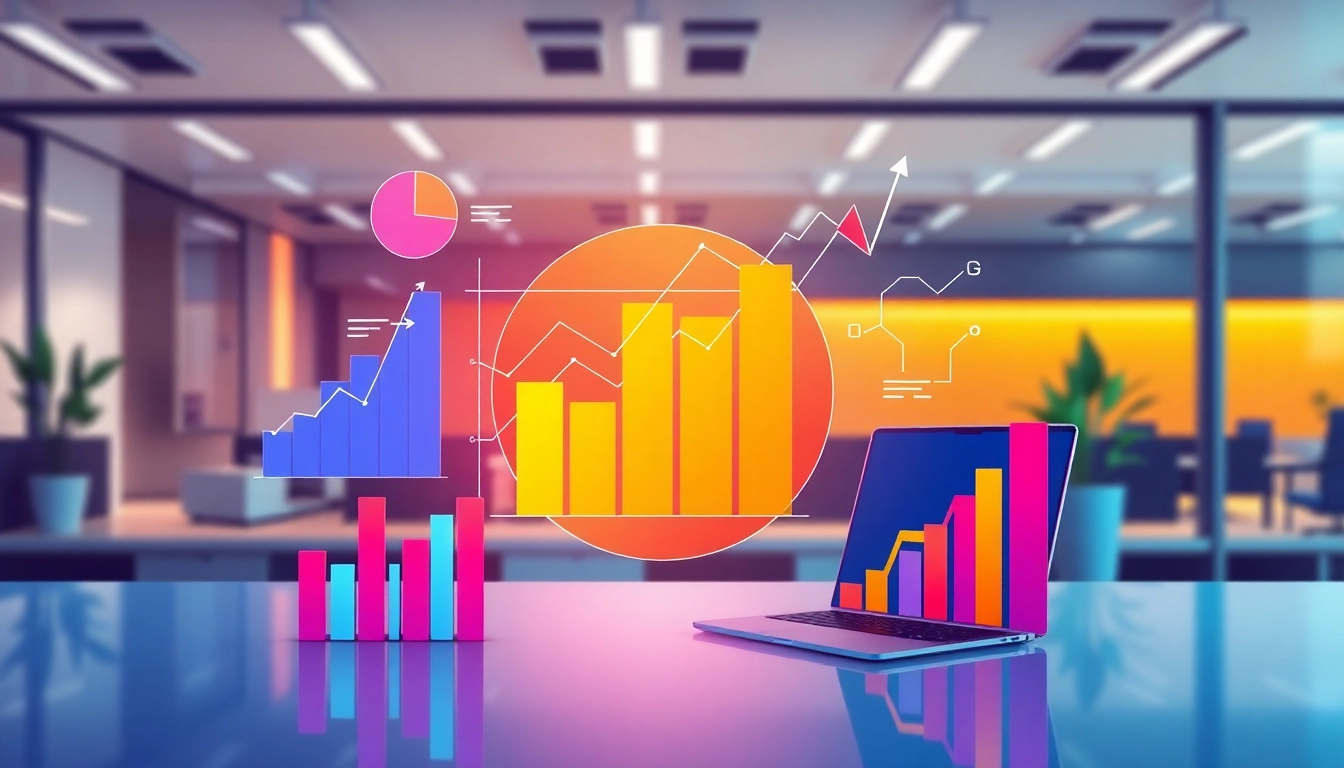
Understanding the Basics of Data Analytics
In today’s increasingly data-driven world, the ability to analyze data effectively can make or break a business. Data analytics involves examining datasets to draw conclusions about the information they contain. This process allows organizations to make informed decisions based on statistical analyses, fostering a culture of evidence-based decision-making. For more information on enhancing your analytical capabilities, you can explore https://www.informaticsview.com. Moving forward, let’s delve deeper into the fundamental aspects of data analytics.
What is Data Analytics?
Data analytics refers to the process of exploring datasets to uncover patterns, correlations, and trends that can inform business strategies. By leveraging statistical techniques and specialized software, analysts can convert raw data into valuable insights. Data analytics can take many forms, including descriptive analytics, which describes past performance; diagnostic analytics, which explains why something happened; predictive analytics, which forecasts future outcomes; and prescriptive analytics, which recommends actions to achieve desired results.
Importance of Data Analytics in Business
Data analytics is critical for businesses, as it empowers them to understand market trends, customer preferences, and internal processes. With an analytical approach, businesses can:
- Make Informed Decisions: Analytics provide the foundation for making evidence-based decisions that align with market demands.
- Enhance Operational Efficiency: By analyzing workflows and processes, businesses can optimize their operations to reduce costs and improve productivity.
- Improve Customer Experience: Data-driven insights help organizations tailor their offerings to meet customer needs and preferences, increasing satisfaction and loyalty.
- Identify New Opportunities: Analytics enables businesses to uncover market gaps and emerging trends, allowing for strategic growth initiatives.
Key Terminology You Should Know
Before diving into data analytics, familiarize yourself with key terms:
- Big Data: High-volume and high-velocity data that is difficult to process using traditional data-processing software.
- Data Mining: The practice of analyzing large datasets to discover patterns and extract valuable information.
- Data Visualization: The representation of data in a visual format, making it easier to spot trends and insights.
- Machine Learning: A subset of artificial intelligence that involves training algorithms to learn from and make predictions based on data.
Tools and Technologies for Effective Analysis
With a multitude of tools available for data analytics, choosing the right technology for your business needs is crucial. Various tools cater to different aspects of the analytical process, from data collection to visualization.
Top Software for Data Analytics
Here are some popular tools widely used in the data analytics field:
- Tableau: A powerful data visualization tool that enables users to create interactive and shareable dashboards.
- Google Analytics: A web analytics tool that tracks and reports website traffic, providing insights into user behavior.
- R and Python: Programming languages that are highly regarded for statistical analysis and data visualization.
- Microsoft Power BI: An analytics tool that transforms data into visually immersive and interactive insights.
Choosing the Right Tools for Your Needs
When selecting tools for data analytics, consider the following factors:
- Business Requirements: Identify what you want to achieve with data analytics and choose tools that align with those goals.
- User-Friendliness: Opt for tools that are accessible, with intuitive interfaces that allow non-technical users to derive insights.
- Integration Capabilities: Ensure the software can integrate seamlessly with your existing systems and data sources.
- Cost and Scalability: Evaluate your budget and consider whether the solution can scale as your data needs grow.
Integrating Data Analytics with Existing Systems
Successful integration of data analytics tools into your business processes involves:
- Centralizing Data Sources: Collect data from various departments into a centralized database, which simplifies data access and ensures consistency.
- Training Staff: Provide necessary training for employees on how to utilize new tools effectively.
- Establishing Clear Protocols: Develop guidelines on how to collect, manage, and interpret data to maintain quality and security.
Best Practices for Data Collection
The effectiveness of data analytics significantly depends on the quality of the data collected. Employing best practices ensures accurate and reliable data, leading to insightful analytics.
Methods of Data Collection
There are several methods for collecting data, including:
- Surveys and Questionnaires: Gathering qualitative and quantitative data directly from users or customers.
- Interviews: Conducting one-on-one discussions to gather in-depth insights on particular topics.
- Web Scraping: Extracting data from websites, which can be useful for market research.
- Transactional Data Logging: Capturing data from customer transactions to analyze purchasing behaviors.
Ensuring Data Quality and Accuracy
To maintain high-quality data, adhere to the following tips:
- Regular Audits: Conduct periodic reviews and audits of your data to identify inaccuracies and duplicates.
- Data Validation: Implement validation rules at the point of entry to minimize errors during data collection.
- Invest in Training: Equip your team with the skills to recognize quality data and the importance of accuracy in data collection.
Legal and Ethical Considerations in Data Collection
Understanding the legal and ethical landscape surrounding data collection is critical. Key considerations include:
- Compliance with GDPR: Ensure that your data practices comply with regulations such as the General Data Protection Regulation, which governs how personal data should be handled.
- Transparency: Be open with stakeholders about how their data will be used, ensuring they can make informed decisions.
- Informed Consent: Always seek consent from individuals before collecting their data, making them aware of their rights.
Interpreting and Visualizing Data
Once data is collected and cleaned, interpreting and visualizing it effectively is key to extracting meaningful insights.
Effective Data Visualization Techniques
Visualizing data helps convey complex information in an understandable manner. Here are some techniques to consider:
- Bar Charts: Useful for comparing categories or groups side-by-side.
- Pie Charts: Effective for displaying the composition of a whole.
- Line Graphs: Ideal for showcasing trends over time.
- Heatmaps: Excellent for representing data density in terms of location or volume.
Turning Data into Meaningful Insights
To translate data into actionable insights, analysts should:
- Highlight Key Findings: Focus on significant trends and outliers that could impact business decisions.
- Develop Narratives: Create stories around the data to make insights relatable and easier to digest.
- Encourage Discussion: Present findings in a way that promotes dialogue and collaboration among stakeholders.
Case Studies: Successful Data Interpretations
To better understand the impact of effective data interpretation, consider these case studies:
- Company A: By utilizing customer feedback data, Company A revamped its service offerings, resulting in a 25% increase in customer satisfaction.
- Company B: Company B analyzed market trends that led them to enter a new market segment, resulting in increased revenue by 15% within the first year.
- Company C: Leveraging sales data, Company C optimized its supply chain processes, reducing operational costs by 10%.
Measuring the Impact of Your Data Strategy
Evaluating the success of your data analytics strategy is essential for iterative improvement and stakeholder confidence.
Key Performance Indicators (KPIs) to Track
Utilize KPIs to measure the effectiveness of your analytics initiatives. Some vital KPIs include:
- Customer Acquisition Cost (CAC): The cost associated with acquiring a new customer.
- Return on Investment (ROI): Measuring the efficiency of an investment relative to its cost.
- Customer Lifetime Value (CLV): The predicted net profit attributed to the entire future relationship with a customer.
- Data Accuracy Rate: A metric to evaluate the reliability of your data.
Adjusting Strategies Based on Data Feedback
Regularly review and adjust your strategy according to data feedback:
- Addressing Underperformance: Identify areas where performance is lacking and implement solutions.
- Testing New Approaches: Use A/B testing to gauge the effectiveness of various strategies before full implementation.
- Soliciting Stakeholder Input: Regularly engage with stakeholders to gather insights and ensure alignment with overall goals.
Continuous Improvement: Learning from Data Trends
Data analytics is an ongoing journey. To foster continuous improvement:
- Encourage a Data-Driven Culture: Advocate for data use in every decision-making process within the organization.
- Stay Updated on Trends: Keep abreast of emerging data analytics trends and technologies to stay competitive.
- Feedback Loops: Utilize regular feedback to refine your analytical approach and methodology for enhanced results.